With off shoring trend reversing in most countries, on shoring with smart plants are becoming increasingly popular choice. Comparesoft studied the key reasons why manufacturers are adopting cognitive and predictive maintenance strategies in their smart plants.
Why Manufacturers Are Focusing on Cognitive and Predictive Asset Management
Prasanna Kulkarni | Comparesoft Ltd.
World Economic Forum suggests that Manufacturers are going to invest $267 billion in Industrial Internet of Things by 2020. With off shoring trend reversing in most countries, on shoring with smart plants are becoming increasingly popular choice. Comparesoft studied the key reasons why manufacturers are adopting cognitive and predictive maintenance strategies in their smart plants.
#1 Assets that understand their environment
Predictive maintenance involves collecting data from your assets — your plant, equipment and stock — during their lifecycle. Once collected, data in great quantities can be analysed using iterative algorithms. The result — intelligent asset management, personalised and optimised to your operating environment.
By selecting modern assets equipped with sensors and instrumentation, or retrofitting existing plant with the same, huge quantities of data on equipment health and performance can be accessed in real time. The next step is extracting value from the data collected.
In a manufacturing environment, with hundreds or thousands of interconnected pieces of machinery, equipment, tools and consumable parts, the flood of data is far too vast and complex for meaningful human analysis. With advanced analytics and intelligent algorithms, structure is imposed on unstructured data and actionable insights can be identified.
Predictive insight is key to knowing when something will break, cognitive insight gives personnel the steps to follow. Rules of thumb, repairing techniques and maintenance procedures are frequently common knowledge among senior staff, but too often key knowledge is lost with staff turnover or retirements and locked away in personal log books. Manufacturers are adopting two pronged approach to mitigate knowledge risks – 1) They are deploying assets that are can react and 2) They are consolidating their qualitative operational/asset information into a centralised and searchable repository.
#2 Optimising maintenance schedules using controlled failure
Manufacturers are always balancing the cost of failure/unreliability and the cost of maintenance and repair. While the cost of a crucial plant asset failing can be easily estimated — in repair costs, in time a production line is halted, in hours finding and installing a replacement —, the cost of too much maintenance is harder to pinpoint.
Source: Aurecon Group
According to a recent study, up to 70% of spending on preventive maintenance does not affect production uptime or reliability. Routine, time-based preventive maintenance pays minimal attention to asset usage, asset health metrics or environmental factors that could have an effect on asset lifetime. An asset replacement schedule that veers too conservative will result in perfectly functional parts being replaced at unnecessary expense. Rather than relying on rules of thumb or equipment manuals to determine failure rates, predictive insight allows some assets to fail in a controlled environment to establish real failure benchmarks.
For example: Paint guns in a production line are rotated out for scheduled maintenance every 900 hours. This does not mean the paint guns are close to failure — whether the likely failure time frame is 1200 hours, 1500 hours, or 1800 hours makes no difference to the schedule. Loosely bolted connections, too high or low paint pressures, miscalibrations are not taken into account.
When a small, controlled number of assets are allowed to fail, sensor and instrumentation data can be used to identify the signs of an impending failure. An increase in overshoot, a stray vibration or any other moving part failure — Manufacturers prefer to use such signals to carry out predictive maintenance when it is needed.
#3 Centralise information and stop interconnecting issues early
Predictive asset management is used to optimise maintenance schedules for single plant assets, but is invaluable in large-scale manufacturing environments. Complex interdependencies arise due to thousands of interconnecting pieces of equipment experiencing millions of interactions between machinery, users and stock. The complications of one asset can ripple downstream through the production line. With predictive asset management, these ripples can be tracked and their effect analysed.
Manufacturers appreciate that predictive technologies focus on extracting insights from quantitative data points. Cognitive methods, however, involves consolidating, classifying and applying qualitative information about your equipment. Accordingly, Manufacturers prefer consolidating user manual texts, instructional videos, technician insights and technical documents into a centralised system - This means an engineer encountering difficulties in the field has everything on hand he needs to diagnose and fix an issue.
#4 Industry 4.0 requires predictive asset management strategy
We have fully embraced the digital industrial transformation, and we believe in its potential to change the world - John Flannery, CEO of GE.
Even for a pioneer like GE it has taken a few years for the transformation. Making the change from traditional to predictive management can seem like an insurmountable expense, especially for large asset portfolios. The good news is that predictive and cognitive asset management can be implemented on a step by step basis.
Manufacturers prioritise expensive, difficult to repair, time consuming assets where downtime would cause a production bottleneck. Most modern plant comes equipped with SCADA sensors and instrumentation to collect the data, but choosing the right predictive management software for your needs will require consultation with vendors.
Manufacturers expect a learning curve for the first few weeks after adopting predictive management method. They typically have their plant technicians on board with the changes and set up daily, weekly and monthly time windows to manually input any extra data, review results and adjust settings.
About Comparesoft
Comparesoft is an AI-driven asset management software recommender. It is used by Honda, GE, Transport for London, Southern Electric and 10,000 other businesses. For further information, please visit www.comparesoft.com/asset-management-software/
The content & opinions in this article are the author’s and do not necessarily represent the views of ManufacturingTomorrow
Comments (0)
This post does not have any comments. Be the first to leave a comment below.
Featured Product
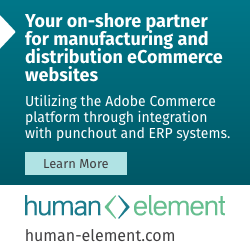