This article will explain the three technologies, that, based on Boston Consulting Group’s Quality 4.0 study – will be deployed by most manufacturers who are planning to improve their quality management.
Readers Choice 2020: Quality 4.0: The 3 Most Important Technologies for Manufacturers
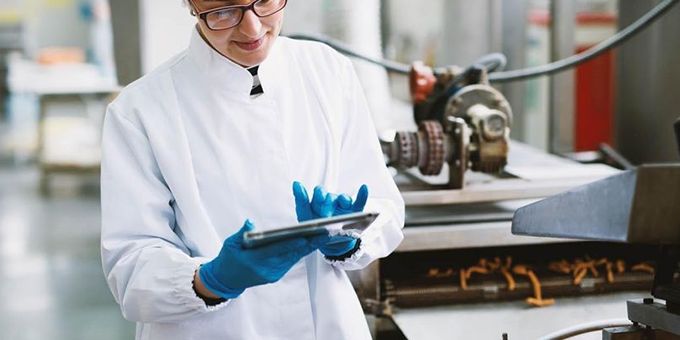
Mikko Nurmimaki | Grip Agency
Industry 4.0 is a buzzword hard to avoid if you are living on the planet Earth! Quality 4.0, on the other hand, is a less-hyped concept to define the technologies, practices, and processes needed to transform quality management for the better. This article will explain the three technologies, that, based on Boston Consulting Group’s Quality 4.0 study – will be deployed by most manufacturers who are planning to improve their quality management. These are Predictive Quality Analytics, Machine Vision Quality Control, and Standard Operational Procedure (SOP).
What is Quality 4.0?
The tremendous ongoing digital transformation – currently dubbed Industry 4.0 – is profoundly changing manufacturing, processing, and production industries. The term Industry 4.0 encapsulates all the rapidly evolving technologies, processes, and practices that are currently changing the world of manufacturing.
Quality 4.0, on the contrary, is a less-hyped, but quickly emerging concept within the Industry 4.0. It tries to further classify those specific technologies, practices, and procedures that enable manufacturers to develop, manage, and maintain quality standards throughout their supply chains.
Quality 4.0 defines the approach that manufacturers can take when infusing new technologies. This includes machine learning, predictive analytics, IoT, big data and cloud computing – with traditional systems for managing quality – such as quality management system (QMS) software – to drive continuous improvement and improve overall business performance.
Digital technologies that are accompanied by smarter practices and procedures can help to improve quality in various ways. For example, companies can monitor processes and collect data in real-time and apply analytics to predict quality issues and maintenance needs. Digital tools also enable people to do their jobs faster, better, and at a reduced cost.
Quality 4.0 isn’t only affecting things that happen inside a factory – it encompasses the entire supply chain – from R&D, to procurement, manufacturing, logistics and sales, to services and after-sales, and other corporate functions - up to the administration and management.
Quality 4.0 use-cases (Image credit – Boston Consulting Group)
Learnings from the Quality 4.0 study
A recent Quality 4.0 study by Boston Consulting Group (BCG), in partnership with ASQ and Deutsche Gesellschaft für Qualität (DGQ), was undertaken to better understand technology's role in quality management transformation. The study focused on the opportunities, and common challenges and technologies related to quality, and how to enhance traditional quality management best-practices.
According to the report, nearly two-thirds of manufacturers believe that Quality 4.0 will significantly affect their operation within the next five years. However, only 16% say their company have begun implementing Quality 4.0 practices, and 63% have not even reached the planning stage!.
While companies recognize that Quality 4.0 can create substantial value, only a few have defined a detailed strategy in place, and already launched an implementation program. Success with Quality 4.0 can only be achieved with a multifaceted approach, which – in addition to the technological issues, also includes a full range of strategic and cultural practices.
The Three Quality 4.0 Technologies in Manufacturing
What are the key enabling technologies behind Quality 4.0? The following chapter of this blog gives an easy-to-understand description of the three most important quality-improving technologies in manufacturing. This is one of the sectors along the complete supply chain, as defined by the BCG study.
Predictive Quality Analytics
Predictive Quality Analytics is a tool used by manufacturers to forecast the quality of the products, components, and materials, that are already in the production process.
Predictive Analytics begins with cleaning, formatting, and analyzing the massive amounts of data collected along the production process. Statistical algorithms and Machine Learning are then applied to the data to extract useful insights. These insights can enable manufacturers to reveal useful correlations between critical variables, recognize data patterns, detect anomalies, and predict future outcomes and trends. It can address the root causes of problems in advance—before any quality issues occur.
Predictive Quality Analytics allows manufacturers to identify abnormal events and root causes, which may lead to reduced quality output – affecting products, components, and materials.
A case in point – Predictive Analytics improving Quality in Battery Cell Production
The eLab Battery Cell production plant (Image credit – Elisa Smart Factory)
The electromobility research center at the Aachen University in Germany recently studied whether predictive quality analytics can be used to increase the manufacturing yield for battery cell manufacturing. In the electric vehicle sector, the battery cell production quality is a major issue - the average scrap rate can be as high as 15%. This increases the costs of electric vehicles, reduces battery production output, and wastes precious raw materials. Costs rise.
The eLab research team, together with Elisa Smart Factory's data scientists, embarked on the search for a solution for higher production quality. They found a solution to the problem. By using predictive analytics – the work started with understanding the manufacturing process from the data perspective through data exploration and using process mining techniques.
Statistical algorithms and machine learning were then applied, to determine data patterns that predicted quality problems further down the line in the process.
As a result of the project, the teams were able to lower the scrap rate of battery cells by 16 percent, by predicting the quality of the battery cells early in the production process!
Machine Vision Quality Control
Machine vision quality control in PCB manufacturing.
In a high-volume, automated process, manual quality inspection is costly and slow. So-called sampling inspection, i.e., determining the quality of an entire production batch by analyzing a small portion of the production is, however, due to the increasing quality requirements, not a scalable solution anymore. It is time-consuming.
This is where Machine Vision and Deep Learning come into play, into the new factory quality control. These technologies enable an automation of inspection—for every product on the line, with consistent and accurate inspection results. Machine vision can remove the human variable from the equation and deliver consistent results, 24/7.
2D vs. 3D inspection
The vast majority of machine vision applications in manufacturing are based on two-dimensional (2D) imaging. It is a rapid and robust method for automatic inspection and offers analysis for applications - such as barcode reading, label orientation, and printing verification.
The three-dimensional (3D) Machine Vision is most commonly used for inspection and the measurement of complex, three- dimensional, free-formed surfaces. Several 3D imaging techniques exist – these include the ‘time of flight’ technique, laser triangulation, stereo vision, light stripe projection, shape from shading, and white light interferometry.
However, the most common method is triangulation, based on laser-scanning by using the motion of the product in the imaging process.
Why is Deep Learning Needed in Visual Quality Control?
Machine Vision cameras are not enough for quality control in manufacturing. All quality defects cannot be identified in the same way. In fact, defining how to identify a defect based on an image can be an incredibly complex task. Even trained professionals can miss tiny details, hinting a quality defect. On the other hand, certain clues may mislead a risk-averse human to discard a product within the limits of acceptable quality. Deep Learning techniques can be used to teach the Machine Vision Quality Control system to detect different types of defects systematically based on images.
Deep Learning involves feeding the Machine Vision Quality Control system thousands of images and training it to learn what’s acceptable quality, and what’s not, and refining these results continuously.
Standard Operational Procedure (SOP)
Standard Operating Procedure (SOP) mobile application.
A standard operating procedure (SOP) is written, step-by-step set of instructions that describe how to perform a routine activity. An SOP can be used to provide instructions for manual and automated tasks, and also functions as a guide for safe work practices. Employees should complete them in the same way, each time so that operations remain consistent.
Benefits of SOPs in Manufacturing
An SOP can help manufacturers to establish consistent, working practices across organizations, maintain a high level of quality, ensure working efficiency and safety, avoid miscommunication and help to prevent failure to comply with industry regulations. It can streamline the production process, while minimizing the risk of errors.
How does a good SOP look like?
An SOP Compliance Template checklist should always be brief, easy-to-understand, and unambiguously worded. Action-steps should be easy to follow. A good standard operating procedure should clearly outline the steps and inform the employees of any safety concerns. SOPs can enable unified training for new employees. They should also be updated periodically to ensure they stay relevant to the current needs of the organization.
How Can Manufacturers Streamline SOPs?
Modern enterprise content management (ECM) platforms allow manufacturers to implement SOPs with advanced features, such as robust access control, centralized version control, easy creation and modification; and management of the procedures.
Role-based training programs, workflows, and task assignments make sure that all employees are following the right procedures, task lists, and training. Electronic signatures can be used to provide evidence and verification of completed tasks. Smartphone SOP-applications now make paper printouts, Excel tables, and ink signatures obsolete, and the total SOP management more efficient.
An SOP can be turned from a mere step-by-step task list to a proactive work order application by using advanced process monitoring and automation. This can evoke timely work orders for tasks listed in an SOP – e.g., order a spare part and provide the right SOP for the required maintenance activity.
In Conclusion
In addition to these top three technologies, the Quality 4.0 study also raised other manufacturing applications – such as automatic root cause analysis, machine-to-machine communication for the self-adjustment of parameters, real-time process simulations, and more.
Quality 4.0, however, is not only about technology. It is a new way of managing quality. Digital tools, coupled with more advanced practices and smarter processes, can now enable quality teams to deliver high-performing products consistently to customers. This can be done while maintaining better safety, higher internal efficiency, and by embracing ecological and sustainable operations.
About Mikko Nurmimaki
Mikko Nurmimaki is a technology marketing specialist with 15 years of experience from world-class brands such as Nokia, Ericsson, Spirent, and more. Currently, his company Grip Agency provides international tech companies with marketing and PR services. Mikko is also the founder and editor of the wearables blog, Smart Ring News.
The content & opinions in this article are the author’s and do not necessarily represent the views of ManufacturingTomorrow
Comments (0)
This post does not have any comments. Be the first to leave a comment below.
Featured Product
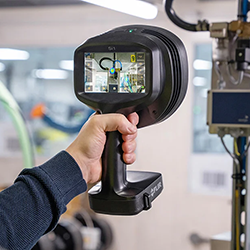