Big data is transforming a variety of sectors, ushering them into the era of Industry 4.0. However, having access to raw data and knowing what to do with it are at completely different ends of the digitalization spectrum.
The Challenges of Smart Manufacturing
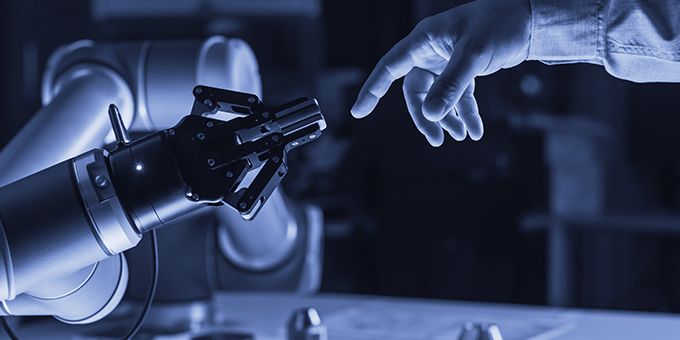
Martin Thunman, CEO and Co-Founder | Crosser Technologies
To help manufacturers understand, and overcome, some of the challenges associated with smart manufacturing, Martin Thunman, CEO and Co-Founder of leading low-code platform for streaming analytics, automation and integration for industrial IoT, Crosser shares his insight.
Before we examine the challenges of digital manufacturing, let’s reflect on industry’s journey. If we consider Industry 1.0 and 2.0 — when mechanization and steam power, followed by the mass use of electrical power came into play — we’re looking at a totally different era of manufacturing. Then the previous revolution, Industry 3.0, began to introduce automated production, IT systems and robotics to the factory floor.
All the previous revolutions have a common connection: their technologies all produced data, of some description. But now, as we enter the Fourth Industrial Revolution, autonomous systems, the IoT and machine learning are equipping manufacturers with the ability to take this data, and make plants more productive, leaner and more cost-efficient.
Possibilities
Having access to data, and more importantly to data analytics, creates a wealth of use cases that can help manufacturers drive value across their business. The starting point for many businesses is getting hold of this data and sending it to a cloud system or data centre for analytics.
The second use case involves factory floor integration, or taking this data and putting it to use. Indicators such as machine health can help form a work order that can be integrated into an enterprise resource planning (ERP) system, equipment health and efficiency can be monitored within the DCS or SCADA system, or machine to machine integration can be used for production optimization, as some examples.
Industry 4.0 technologies are also a driver for advanced automation. Moving beyond previous, more rigid systems of automation, new technologies allow machine to machine automation to take place, in a faster and much more data-driven manner.
The fourth case involves understanding activities along a production line, and creating goals for a machine, process or a complete plant based on data insights. The final use case is the leveraging of machine data for processes beyond the factory floor. For example, supply chain, sales and finance could all benefit from data analytics — it isn’t all about machine health and operations on the shop floor.
The challenges
But there are challenges, and making these use cases a reality can seem difficult to realize – especially if an organization doesn’t have software developers onsite.
According to Actify.com, 33 percent of all data could be useful when analyzed. However, companies only process 0.5 per cent of all data. By incorporating an enterprise data strategy, companies can ensure they are processing useful data and that time is not wasted on the rest.
However, knowing how to manage raw machines can be difficult. It’s important to think of a data plan as the foundation for success — hyped-up technologies like machine learning and artificial intelligence (AI) will come after. Manufacturers should follow three key principles when building a data strategy. Firstly, the strategy needs to be practical and easy to implement across the organization. It also needs to be relevant and specifically tailored to the company’s goals as well as evolutionary and adaptable, to keep up with current trends. Finally, the strategy must be universally applied across the business and easy to update when necessary.
The second challenge is complexity, which comes in multiple layers. Legacy machines standing next to brand new robots, multiple generations of protocols and programmable logic controllers (PLCs), fragmented operational technology (OT) systems and segmented networks are just some of the drivers of complexity that create a large volume of varying data. How do you manage this data flood?
Managing data is hard for many manufacturers, but when combined with a lack of digital resources, there are further challenges to overcome. A report by the National Skills Coalition found that more than one-third of the manufacturers it surveyed have limited or no digital skills, while just 29 per cent possess the advanced skills necessary to be most adaptable to changing technology.
Currently, there are over 1.3 million openings for software roles on LinkedIn — illustrating a burgeoning demand that isn’t being filled. Companies might believe that their IoT challenges can be solved by hiring a developer, but they’ll struggle to make this happen overnight.
The solution
Instead, empowering the existing team is critical. At Crosser, we believe smart tools are key to supporting existing teams and helping them to innovate. Our response to the aforementioned challenges is built around three key principles.
The first is self-service simplicity, which is enabled by an approach that we refer to as “low-code”. This method is trending across industry, to facilitate innovation without reliance on software developers. With pre-built modules, which can be dragged and dropped to develop specific use cases, and visual design flows that facilitate IT/OT collaboration, teams without software development skills can work together to bring Industry 4.0 into their facilities.
The Crosser library features pre-built building blocks designed for any request or ambition. By selecting relevant analytics modules and enterprise connectors, companies can collect their data, attach meaning to it, apply intelligent logic and create clear workflow actions.
Just having a means of processing data isn’t enough. Businesses also need an architecture that addresses the entire data lifecycle. Integration platforms have evolved from the old and slow enterprise service bus (ESB) solutions through integration platform as a service (iPaaS) models to the next generation platforms, often referred to as hybrid integrations.
Having a hybrid architecture delivers full flexibility to deploy processing and integration nodes either on-premise, on the edge, in a cloud or data center. Offering the best of both worlds, businesses can deploy low-code solutions anywhere, but are still able to handle them on a single platform.
Deployment of Industry 4.0 technologies may be growing, but that doesn’t mean it’s without challenges. Having the right people, knowledge and infrastructure to make data management a reality can seem out of reach. But implementing a single, reliable and user-friendly Edge Analytics Platform can bring the latest technologies to any facility — with or without software developers.
The content & opinions in this article are the author’s and do not necessarily represent the views of ManufacturingTomorrow
Comments (0)
This post does not have any comments. Be the first to leave a comment below.
Featured Product
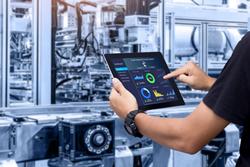