Data analytics is a powerful tool for manufacturers, which involves applying mathematical techniques to extract valuable insights from data. This can help to improve systems, understand trends, and increase efficiency across various industries.
What is Data Analytics?
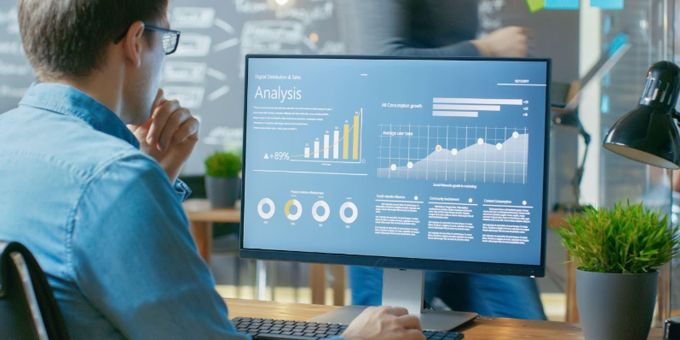
David Bowles | Promethean Software Services
Data analytics is a powerful tool for manufacturers, which involves applying mathematical techniques to extract valuable insights from data. This can help to improve systems, understand trends, and increase efficiency across various industries. For manufacturers, critical data is often scattered and disorganized, making it difficult to extract meaningful insights. Therefore, it is crucial for companies to store their data effectively for analysis to be useful.
Manufacturers can track a variety of quantities, such as customer visits, sales, key performance indicators (KPIs), machine diagnostics, and reviews. Applying analytics to this data can provide endless benefits, including improved decision-making, a greater understanding of customer behavior, and enhanced efficiency. It is essential for manufacturers to have easy access to their data to stay competitive in today's digital age.
Data analytics can be classified into four types: descriptive, diagnostic, predictive, and prescriptive analytics. Each type of analysis serves a specific purpose and can provide insights that help manufacturers to manage their businesses effectively. With the right data analysis approach, manufacturers can gain a clear competitive advantage in their industry.
The Different Types of Analytics
Descriptive Analytics involves answering questions about what has happened in their business operations. This type of analytics is primarily used to run reports, compile raw data, and provide answers to questions such as how much, how many, or when past events took place. Reporting questions could include inquiries like:
- What was our busiest month?
- Which team member had the highest sales last week?
- How many units did we produce in a specific period?
Descriptive analytics is a valuable tool to gain insights into a company's current health and performance. However, it is only the first step in data analysis and should not be the only method used. While descriptive analytics helps answer questions about past events, using this method alone to predict and explain events is not always sufficient. To gain deeper insights, manufacturers should consider using more advanced forms of data analysis such as diagnostic, predictive, and prescriptive analytics.
Diagnostic Analytics involves answering the question of why it happened. This is achieved by finding connections between different types of data. Diagnostic Analytics is an involved process that requires large quantities of data, as analytics alone cannot always determine if two events are causally related. Understanding whether one event caused another often requires additional information.
To illustrate the concept of Diagnostic Analytics in the manufacturing industry, let's consider the relationship between machine downtime and production output. While these two variables are related, simply observing that production output decreases when downtime increases is not enough to establish causation. Instead, a better explanation for this relationship would be that both downtime and production output are influenced by factors such as machine maintenance, operator performance, and raw material quality. Understanding the causal relationship between these variables requires more advanced statistical methods and a large amount of data.
For example, if the data analysis shows that machine downtime increases when maintenance is delayed or when operators are not properly trained, then addressing these underlying issues could reduce downtime and increase production output. By using Diagnostic Analytics to identify the root cause of problems, manufacturers can make more informed decisions to optimize their operations and improve production.
Despite its complexities, Diagnostic Analytics is a powerful tool that should not be overlooked. Once the causal relationship between variables is established, the trends of one dataset can inform the trends of the other and provide significant predictive power. Diagnostic Analytics is a necessary first step in performing advanced analytics and is particularly effective when used in conjunction with Predictive Analytics.
Predictive Analytics is a powerful tool that helps answer questions about what might happen in the future. By using data from the past, this analysis can make predictions about a wide range of events. Some examples of questions that can be answered by Predictive Analytics in manufacturing include:
- What will sales look like next month?
- When will our busiest day be next week?
- When is a particular machine likely to break down?
One example of Predictive Analytics for manufacturers could be predicting machine failures. By analyzing data from machines, such as sensor data, operators can identify patterns that lead to breakdowns. Using this information, manufacturers can build a predictive model that factors in machine age, usage, and other relevant factors to predict when a machine is likely to fail.
This predictive model can then be used to inform maintenance schedules, allowing operators to schedule repairs or replacements proactively, rather than reactively. By using Predictive Analytics to anticipate and address machine failures, manufacturers can minimize downtime, reduce repair costs, and improve overall efficiency. This not only saves money but can also prevent unexpected production disruptions and maintain customer satisfaction.
While Predictive Analytics is a valuable tool, it is important to approach it with a level of skepticism and recognize its limitations. There are instances where the predictions may not be reliable, such as during periods of chaos or when a system is largely determined by randomness. In such cases, it can be challenging to create accurate models and predictions.
Despite its limitations, Predictive Analytics is an invaluable resource for manufacturers, and decision-makers should aim to develop the skill of knowing when to trust its predictions. When predictive analytics is working well, and there are methods for testing, it can provide significant benefits, including better decision-making, improved efficiency, and increased profitability. As such, it should be an essential tool in any manufacturer's decision-making toolkit.
Prescriptive Analytics involves answering the question of what should be done next. This type of analytics represents the forefront of data science and is the most complex form of analytics mentioned so far. Prescriptive Analytics goes beyond other forms of analytics by utilizing all available data to determine the best course of action.
To achieve this, machine learning algorithms are commonly used as a core method for problem-solving. While this approach may seem impenetrable, significant progress has been made to lower the barrier to entry for manufacturers. However, Prescriptive Analytics still requires considerable knowledge to be effective.
Prescriptive Analytics can be understood through the example of a computer chess game. In this case, Prescriptive Analytics would involve providing recommendations on the next best move for the player. This is accomplished by analyzing the configuration of pieces on the board (Descriptive), calculating the possible/probable moves for the opponent, and then evaluating the favorability of each possible move for the player (Predictive).
The system then synthesizes this information to suggest the best move possible for the player, which is the Prescribed move. By using this type of Prescriptive Analytics, the player can make a more informed decision about their next move, which can improve their chances of winning the game.
An example of Prescriptive Analytics more for manufacturers could be optimizing production schedules to reduce costs and increase efficiency.
By utilizing data from various sources, such as machine data, operator performance data, and supply chain data, Prescriptive Analytics can generate recommendations on how to optimize production schedules. For instance, the analytics could determine the optimal order in which to schedule production jobs based on factors such as setup times, machine availability, and material availability.
Using Prescriptive Analytics, manufacturers can make more informed decisions about how to optimize their production schedules, which can help to reduce downtime, lower costs, and improve efficiency. While this type of analytics requires a significant investment in terms of time and resources, the benefits can be significant for manufacturers who are looking to maximize their operations and stay competitive in their industry.
Why is Analytics Necessary?
For manufacturers, Analytics is a necessary tool for maximizing profits and efficiency. From basic reports to complex machine learning algorithms, companies of all sizes are adopting analytics as a cost-effective way to achieve their business goals. Analytics serves as a safeguard against biased thinking and misleading intuition, elevating the quality of information available for decision-making.
One of the significant benefits of analytics is its ability to mitigate logical fallacies that can plague decision-makers. Common logical fallacies that could lead to traps include confirmation bias, survivorship bias, observational selections, probability of small numbers, and cherry-picking. By understanding these fallacies and relying on non-biased analysis, manufacturers can make more informed decisions and avoid costly mistakes.
As Carl Sagan notes in his book, "The Demon-Haunted World," science is always reminding us that we are new at this and might be mistaken. This same principle applies to decision-making in manufacturing, where intuition alone may not be sufficient. However, when intuition is supported by the clear sight that analysis provides, manufacturers can make decisions that can enhance their operations, improve efficiency, and ultimately increase profitability.
How to Start Doing Analysis?
To start doing data analytics as a manufacturer, you should follow these steps:
Identify your business goals: Determine what your business goals are and identify the areas where you want to apply data analytics. For example, you may want to improve your production efficiency, reduce downtime, optimize your supply chain, or improve product quality.
Collect data: Identify all relevant data that pertains to the area you want to improve. This can include data from sensors, machines, and other devices, as well as data from your ERP, CRM, and other systems.
Store data: Store the collected data in a central location, such as a data warehouse, to make it easier to access and analyze.
Clean data: To ensure it is accurate, consistent, and complete. This involves establishing data governance policies and processes and implementing data validation and cleansing processes to identify and correct data errors and inconsistencies.
Analyze data: Use data analytics tools and techniques to analyze the data and identify patterns, trends, and insights. This can include using statistical analysis, machine learning, or other techniques.
Visualize data: Visualize the results of your analysis in a way that is easy to understand and interpret. This can include using charts, graphs, and dashboards.
Take action: Use the insights gained from your data analysis to make data-driven decisions and take action to improve your business processes.
Monitor progress: Continuously monitor your progress and refine your data analytics approach to ensure that you are achieving your business goals.
Overall, starting to do data analytics as a manufacturer requires careful planning, data collection, analysis, and action. It can be helpful to work with a data analytics consultant or team to guide you through the process and ensure that you are getting the most value from your data.
Benefiting from Analytics
For manufacturers, the benefits of Analytics cannot be overstated. Analytics is vital to enhancing the quality of decision-making and ensuring the longevity of your business. However, performing effective analysis is not just a mathematical discipline, it's a mindset. Manufacturing professionals must cultivate a mindset of thinking in terms of data that can be gathered and analyzed when formulating questions for analysis.
While it can be difficult to see the relationship between data and the questions it can answer, cultivating a sense of curiosity is a vital ally in maximizing the benefits of analysis. There will always be more to learn, and by fostering a sense of curiosity, manufacturers can continuously improve their operations and optimize their decision-making processes.
However, it's important to be mindful of the fact that not all analysis leads to profitability, and it's easy to get carried away. To avoid this, manufacturers should set clear goals for the questions they want to answer and establish a plan for how they will accomplish them. Setting deadlines can also help to ensure that the analysis stays on track and focused on achieving the desired results.
In summary, for manufacturers to benefit from Analytics, they need to cultivate curiosity, be data-driven, and set clear goals and plans for their analysis. By doing so, manufacturers can make more informed decisions that can improve their operations, enhance efficiency, and increase profitability.
About David Bowles
David Bowles is a data analyst and a physics doctoral candidate at the University of Kentucky. He has a Masters degree in Physics and Bachelor degrees in Mathematics and Physics from the University of Kentucky. He has 10+ years of experience in data collection, data analysis and mathematical modeling for several large scale nuclear and particle physics collaborations. He provides practical and actionable insights on how to use data analytics to improve decision making, optimize processes, and increase profits. To learn more about David Bowles and his work, visit PrometheanSSI.com.
The content & opinions in this article are the author’s and do not necessarily represent the views of ManufacturingTomorrow
Comments (0)
This post does not have any comments. Be the first to leave a comment below.
Featured Product
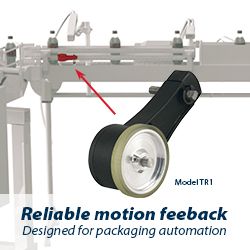