By leveraging Generative AI, manufacturers can initiate a wide array of use cases in areas such as customer service, product and quality control, supply chain, logistics, predictive maintenance, marketing and sales, finance, compliance and legal, and research and development
Unleashing the Potential of Generative AI for Unprecedented Success in the Manufacturing Industry
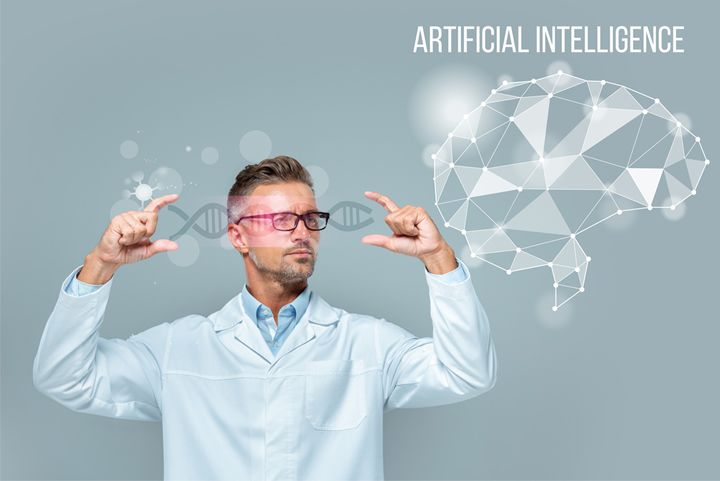
Q&A with Mr. Amit Gautam, CEO | Innover
Tell us about yourself and Innover.
In my role as Chief Executive Officer for Innover, I am responsible for all aspects of the company’s product & services strategy & execution, as well as its financial performance and growth. Innover was started with a vision - to become the world's leading digital transformation firm with an unyielding focus on both our customers and employees. Headquartered in Alpharetta, Georgia, our mission is to help our clients become connected, insight-driven enterprises. With an unwavering commitment to growth and innovation, we've been at the forefront of pioneering outcome-driven transformations and delivering exceptional experiences across the supply chain and manufacturing industries.
Across the globe, we cater to a diverse clientele, including 65+ Fortune 1000 companies. Our presence spans across 15 locations, with delivery centers across the USA, Canada, Mexico, and India. With a powerhouse team of 1500+ Innovators, adept in AI, ML, Data Science, Software Engineering, Advanced Analytics, Design Thinking, Metaverse Technologies, and RPA, Innover pioneers excellence and ensures unmatched customer success. Our three studios - Data & Insights, Digital Experiences, and Digital Operations – work in tandem to deliver successful digital transformation projects at scale. These digital studios build a connected ecosystem driven by insights, powered by cutting-edge technology, and accentuated by experiences to deliver 360° impact and ROI-driven outcomes for our customers.
What are the key challenges and barriers manufacturers face today?
Manufacturing companies have a wealth of data originating from diverse sources, including production data, machine sensor data, equipment and maintenance data, distributor and partner data, and real-time sales and customer data. It is crucial for them to collect, integrate, analyze, and manage these diverse data streams at an enterprise level. As manufacturing operations grow, the need to scale data infrastructure to accommodate larger volumes and increasing complexity becomes a pressing challenge. Amidst all this, extracting real-time insights from fragmented and siloed data becomes a necessity to drive visibility across manufacturing operations.
Another challenge is identifying and solving the ‘right’ problems using the ‘right’ technologies to spearhead digital transformation endeavors. I have never had a customer approach me saying that they want to implement a certain technology; instead, they typically describe the problem at hand. For example, a manufacturer might be looking for ways to strengthen their demand forecasting systems. With a clear goal in mind, we suggest and implement the right blend of technologies to achieve desirable outcomes, bolster their operations, and amplify ROI.
In your view, how can Generative AI help manufacturing organizations?
Generative AI is poised to bring about a remarkable transformation in the manufacturing industry, and we've noticed a significant surge in interest from our manufacturing clients. They are genuinely excited about harnessing the potential of Generative AI to elevate their operations and achieve heightened efficiency. This cutting-edge technology has the potential to empower manufacturers to make the most of their data, effectively supercharging their teams to access real-time insights, boost productivity, and yield measurable results. The technology can understand and interpret natural language queries and user prompts, delivering precise, context-aware answers in just seconds. It can effortlessly streamline tasks such as document search, extraction, and summarization, and support conversational question-answering capabilities. Moreover, its advanced algorithms can identify patterns, trends, and anomalies that might be challenging to discover through manual analysis, simplifying the process of finding valuable insights.
Generative AI also revolutionizes operations by unleashing unprecedented efficiency and performance across the entire spectrum of manufacturing lines. By leveraging Generative AI, manufacturers can initiate a wide array of use cases in areas such as customer service, product and quality control, supply chain, logistics, predictive maintenance, marketing and sales, finance, compliance and legal, and research and development - ensuring the lights never go off and engines keep running. For instance, Generative AI models can simulate production scenarios, accurately predict demand, and optimize inventory levels. By analyzing past production trends, customer demand, and other historic data, these models can help manufacturers strike the right balance between production and inventory, reducing excess stock and minimizing shortages.
Given the multitude of models available in the market, how can manufacturers employ the most suitable model aligned with their business need?
Manufacturing organizations have their own distinct operational nuances, industry insights, and historical data that cannot be adequately captured by a one-size-fits-all model. To extract the most out of their Generative AI investments, manufacturers face the critical challenge of selecting the most suitable model that aligns with their specific needs. One approach is to begin by leveraging off-the-the-shelf models, also referred to as Foundation Models. While these generic models offer a broad range of capabilities, they often fall short when it comes to meeting the specific demands of the manufacturing businesses, potentially impeding performance and impacting customer experiences. Essentially, what is gained in terms of speed and simplicity is often lost in terms of control and customization.
On the other hand, building and managing custom models from scratch grants organizations complete autonomy, but can be a complex and costly undertaking. Training these models requires access to large datasets and specialized infrastructure, which can pose challenges for many manufacturing organizations, especially in the initial stages of AI adoption.
Given the above limitations, fine-tuning emerges as a recommended method to enhance the value and impact of AI models. Through fine-tuning, manufacturing organizations can leverage their existing domain-specific data and combine it with the capabilities of the foundation model, - unlocking new frontiers of performance. Fine-tuning offers organizations the flexibility to choose and enhance models while also enabling the option to switch models based on continuous monitoring and evaluation. This allows manufacturers to strike a balance between customization, efficiency, and cost-effectiveness while maintaining control. In our view, companies that fine-tune their foundational AI models in the context of their specific business ecosystem will achieve maximum differentiation and ROI in the future.
How can manufacturing organizations succeed in their Generative AI journey?
Early adoption of Generative AI can offer manufacturing organizations a strategic edge, although the specific approach and strategy can differ among companies, and even within different departments of the same organization. To jumpstart their Generative AI initiatives, manufacturers will have to first decide whether to pursue large-scale deployments or initiate smaller-scale experiments. This initial decision will chart the way forward.
The journey commences as manufacturers identify the ‘right’ use cases that promise substantial impact and competitive edge. They must evaluate whether the chosen solutions align with their long-term goals and overall vision. Following this decision, manufacturers need to assess critical factors such as budget, scale and quality of available data, privacy and security requirements, network latency, and anticipated request volume. The most suitable course of action also depends on the company’s goals and its preparedness to handle risks.
We're in the midst of an exciting journey, where we're actively engaged in multiple Proof-of-Concepts and guiding top-tier clients in the manufacturing industry to turn their investments in Generative AI into resounding successes. To achieve this, we have also launched our proprietary Generative AI Framework- Innosight.ai™. This innovative framework combines the latest Generative AI capabilities, foundation models, and Machine Learning techniques to empower manufacturers to seamlessly integrate AI into their operations and optimize their domain-specific tasks. The framework also provides a range of resources, including solution approaches, accelerators, responsible design frameworks, and industry-specific solutions. Innosight.ai™ stands out by offering holistic enterprise transformation, cutting through the hype and delivering tangible value.
What challenges do manufacturers typically face when adopting Generative AI?
Before embracing Generative AI, manufacturing organizations should thoroughly evaluate their readiness, particularly when they might lack the underlying technology and talent. For instance, if the available data is insufficient or of low quality, the initial focus should be on establishing a strong data foundation before venturing into Generative AI implementation. It is also imperative to have robust data handling and security protocols in place to protect sensitive customer data and comply with privacy regulations. Careful planning, including hardware selection aligned with processing power, memory, and storage needs, and choosing suitable scaling options, is crucial to optimize costs and efficiency.
It is also important for manufacturers to understand Generative AI may not be a standalone solution for all their requirements. The full potential of Generative AI will be realized when it converges with technologies like voice assistants, speech-to-text, and robotic process automation, enabling real-time impact and facilitating autonomous decision-making.
Another crucial challenge for manufacturers pertains to ethical dimensions. They must address four fundamental dimensions: privacy, fairness, bias, and model robustness. It is imperative for manufacturers to not only proactively address potential biases or discriminatory outcomes but also ensure the utmost reliability of their AI models. Overall, manufacturers must establish resilient frameworks and analyze the right applications of the technology to position their businesses for consistent growth, sustained competitiveness, and long-term profitability.
What are some of the top Generative AI use cases that hold relevance for manufacturers in today's business landscape?
Some of the top use cases within the manufacturing sector where we are observing the impact of Generative AI are:
-
Customer Service: Equipping service agents to navigate through vast customer logs and condense bulky documents to glean quick insights to decipher customer intent, effectively categorize support tickets, and promptly resolve escalations.
-
Content Generation: Automating the creation of product descriptions, labels, training videos, marketing assets, data sheets, technical manuals, and more - enhancing accuracy, expanding scalability, and accelerating time-to-market.
-
Predictive Maintenance: Analyzing historical sensor data and equipment performance to anticipate potential faults and failures, enabling proactive maintenance, limiting downtimes, curtailing costs, and expediting operations.
-
Field Force Services: Empowering field service technicians to sift through extensive documents such as safety guides, installation manuals, and product demos to automate and distill insights in mere seconds - reducing operational disruptions, mitigating downtimes, and elevating customer experiences.
-
Supply Chain Visibility: Enabling manufacturers to seamlessly traverse complex supply chain data, encompassing TMS, WMS, order management, logistics, customer data, and more, with a simple prompt - bolstering supply chain visibility and facilitating guided decision-making.
For more information on generative AI use cases and how organizations can harness its benefits while ensuring security, scalability, and repeatability, please refer to this whitepaper.
About Amit Gautam
In his role as Co-Founder and Chief Executive Officer for Innover, Amit Gautam is responsible for all aspects of the company’s product & services strategy & execution, as well as its financial performance and growth. Amit has a relentless focus on Growth and Innovation and holds a strong personal commitment towards “Outcome-Driven” Digital Transformation for businesses. Amit collaborates with the C-suite executives of Fortune 1000 companies and guides them to adopt a digital-first mindset, delivering bold transformations and exceptional experiences. Prior to Innover, Amit worked with firms like GE and Cognizant in various leadership roles. Amit studied Data Science at Harvard and holds a Bachelor's degree in Engineering from India.
The content & opinions in this article are the author’s and do not necessarily represent the views of ManufacturingTomorrow
Comments (0)
This post does not have any comments. Be the first to leave a comment below.
Featured Product
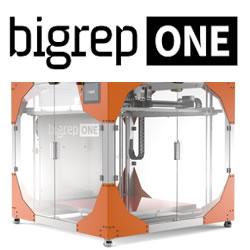