In a recent SparkCognition webinar, “Top 3 Ways AI is Transforming Manufacturing,” Andrea Schmidt and Bhavana Brar explored the topic of Industry 4.0, outlined the topline challenges and opportunities of adopting AI in the manufacturing industry.
Is Industry 4.0 Here? How AI is Transforming Manufacturing Today
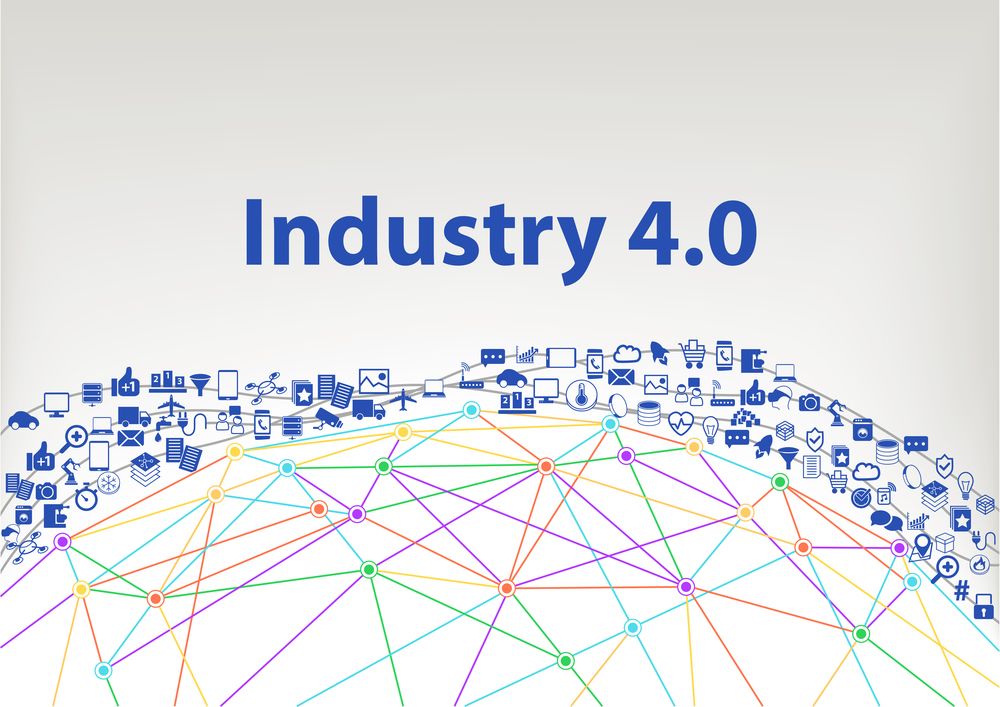
Campbell LeFlore | SparkCognition
In a recent SparkCognition webinar, “Top 3 Ways AI is Transforming Manufacturing,” Andrea Schmidt and Bhavana Brar explored the topic of Industry 4.0, outlined the topline challenges and opportunities of adopting AI in the manufacturing industry, and walked through several real-world use cases in predictive maintenance, prescriptive analytics, and process optimization in quality assurance.
Schmidt is SparkCognition’s Director of Solution Architecture, helping customers understand the technological capabilities of AI and scope out solutions that fit their business needs and organizational requirements. Brar is Senior Director of Aerospace and Defense Industrial AI Programs at SparkCognition Government Systems, overseeing engineering, execution, and customer relationships.
What exactly is Industry 4.0?
What is the Fourth Industrial Revolution, aka Industry 4.0? McKinsey has defined it this way: “Steam propelled the original Industrial Revolution; electricity powered the second; preliminary automation and machinery engineered the third; and cyberphysical systems—or intelligent computers—are shaping the Fourth Industrial Revolution.”
Schmidt explained it as “driven by the next generation of AI-powered analytics and built upon the advancements in Industry 3.0’s real-time data collection and data storage capabilities.”
“The AI layer is what unifies the various operational data silos and provides an interconnected, more holistic approach to manufacturing. With advancements in AI, manufacturers now have the ability to tease out efficiency gains, reduce costs, increase throughput, and improve not just the quality of end products, but also the quality of the human experience on the factory floor.”
Highlighting the difference between Industry 4.0 and the previous era of 3.0., Schmidt drew out three main factors: “One is technological convergence […] the exchange of data between machines, systems, and services is [becoming] more standardized and also secure. Two would be the new ecosystem based on open innovation. And three is systemic remodeling, affecting production consumption and logistics systems.”
Having explained what Industry 4.0 signifies, what evidence supports the case that it has truly arrived? Strong signals from the market indicate that the door to four is standing wide open, and top manufacturers are already crossing its threshold. The market for AI in manufacturing is projected to see the largest growth year over year of any market in 2024, rising at a compound annual growth rate of around 33%. Manufacturers who understand the value AI can provide will be trialing and onboarding platforms and systems into their daily workloads to improve things like efficiency, reduction of waste, and improving workplace safety. According to a recent Deloitte survey, “83% of companies think AI has made or will make a practical and visible impact. Among these, 27% believe AI projects have already brought value to their companies, and 56% think these projects will bring value in 2-5 years.”
Inviting Brar to highlight SparkCognition use cases that showcase transformative gains in manufacturing that pivot on AI-enabled technology applications, Schmidt encouraged listeners to “consider where you are in terms of technology implementation at your own facilities.” What use cases come to mind for your operation that could be enhanced and—that word again, transformed—by extracting insights from data to predict when an asset will need maintenance, address repairs more efficiently with prescriptive analytics, or augment a critical workflow with generative AI? What does Industry 4.0 mean to you?
Industry 4.0 in action: Follow the data, Learn from the data
Brar began by talking about the drivers pushing wider and deeper adoption of AI in manufacturing in the last few years (large amounts of useful digital data; faster, more powerful computers and processors built specifically for AI; and a larger ecosystem of AI algorithms to work with.) “All of these factors have essentially set the stage for manufacturing companies to adopt AI for their day-to-day processes to drive improvements” she said. “And we’ve actually got some solid metrics on how AI has actually helped improve productivity and drive cost down within organizations.”
Noting the challenges and opportunities typical of the manufacturing sector, Brar put reactive maintenance of critical assets at the top of the list.
“So, in other words, you’re addressing equipment and machinery issues only after they’ve occurred. The ramifications of reactive maintenance are many. Some of the obvious ones are one is the cost of the downtime and equipment damage. Delayed maintenance increases the risk of equipment damage, which can further escalate repair costs and extend downtime. And then, I think, most importantly is safety concerns—safety risks to workers in the surrounding environment. So the opportunities for AI for this particular challenge would be the use of AI algorithms for predictive maintenance to analyze the historical equipment data, such as sensor readings and performance metrics, and being able to predict when the equipment is likely to fail. AI-powered monitoring systems can also be used to continuously analyze real-time equipment data to detect anomalies and early signs of potential failures. So this is a huge value add to any manufacturing facility where you have critical assets that could essentially turn out to be a bottleneck for your yearly production. Then also: just being able to use AI to optimize maintenance schedules that are based on factors such as equipment usage, patterns, production schedules, and the criticality of assets.”
Brar also noted inadequate operational visibility of asset health, citing the all-too-common scenario of managers on the factory floor who have only limited insight into the health and performance of their surrounding manufacturing assets: “So again, the opportunity here is the ability of AI to help with real-time monitoring, predictive analytics, and prescriptive recommendations.”
Brar’s third callout was the challenge of data standards (or lack thereof). She explained:
“So what this means is essentially the inconsistent data formats and standards across different systems and departments, making it really difficult to aggregate, analyze, and derive meaningful insights from the data. So you’re trying to integrate data from disparate data sources. It’s very complex and it’s very time-consuming. This slows down the efforts to leverage this data for decision-making and optimization. And, of course, there’s also quality and accuracy issues. A lot of times, the data is manually entered, and you can end up with quality problems and gaps in the data. The opportunity for AI is that the data integration process and the data preprocessing help to standardize all of this data […] so that it’s consumable for the AI models.”
Jumping in, Schmidt pointed out that “the data standardization really is key because it must precede any AI initiative that you’re going to do.” In other words, once your data standards ticket is punched, you can go virtually anywhere with quality data sets to work from.
SparkCognition case studies: Improving workflows and enabling outcomes
At this point in the webinar, Brar transitioned to presenting several case studies where SparkCognition provided transformative value for manufacturers:
- Helping a prime aerospace company ensure uptime of their critical autoclave assets by providing predictive maintenance alerts and real-time equipment monitoring to detect anomalies, plus prescriptive intelligence to guide operators to repair issues faster.
- For another major aerospace company, providing a prescriptive analytics solution to reduce repair turnaround time by leveraging historical test and repair data to inform troubleshooting and fix recommendations, improving operational planning via insights into KPIs, and mitigating impacts of workforce turnover.
- Improving maintenance workflows for an aerospace company challenged by aircraft production and sustainment specifications and requirements written in archaic language—SparkCognition utilized the latest LLM technology to simplify text, reduce duplication, and consolidate documentation, effecting a lower cost to supply base and less maintenance burden within an ITAR compliant environment.
- A truly innovative implementation combining computer vision and augmented reality to scale and operationalize visual inspection in support of quality assurance for parts manufacturing for a leading aerospace manufacturer.
You’ll want to go to the 10:50 minute mark of this information-packed webinar to learn the full details of these powerful case studies, plus key takeaways from our subject matter experts, Schmidt and Brar. You may be surprised to learn the extent to which AI is transforming manufacturing today, led by SparkCognition.
The content & opinions in this article are the author’s and do not necessarily represent the views of ManufacturingTomorrow
Comments (0)
This post does not have any comments. Be the first to leave a comment below.
Featured Product
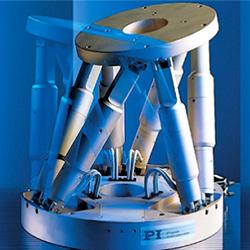