What Does Industry 4.0 Mean for Data Analytics?
In 2011, if you told someone we were entering the Fourth Industrial Revolution, they might have been dubious. A decade later, everyone's talking about integrating smart technology into the manufacturing process. More than two-thirds of companies polled in a recent survey see Industry 4.0 as a top strategic priority, and Google searches for the keyword—which didn't exist ten years ago—are now neck and neck with those for "lean manufacturing."
The opportunities are, theoretically, vast. By 2025, manufacturers and suppliers implementing Industry 4.0 tactics are expected to create $3.7 trillion in value, according to research by McKinsey and the World Economic Forum.
However, we're not there yet. As many as 65% of executives still aren't realizing value from their artificial intelligence and machine learning investments, one recent study found.
Without seeing a payoff, many are understandably cynical. When asked why they hadn't yet rolled out Industry 4.0 solutions on a larger scale, respondents cited the lack of compelling business cases, saying it was hard to justify investments without seeing short-term results. Maybe AI and ML had reshaped the worlds of finance, e-commerce, and adtech—but these executives remained unconvinced that smart technology could do anything for their factories.
They're overlooking a massive opportunity. Behind all the hype, Industry 4.0 is a game-changer, both for data analytics and manufacturing as we know it.
Smart technologies like machine learning help manufacturers turn the data they're already collecting into useful insights, then take action on them. The results are tangible—from increased efficiency and higher quality to cost savings and minimized emissions.
Better data insights
Unlike in the original Industrial Revolution, modern factories have hundreds of sensors collecting data at each point in the production process. The vast majority of manufacturers are already storing this data, and maybe even leveraging some of these datasets for analysis.
But most continue to rely on traditional analytical tools, which can only take you so far. Say you manage a chemical plant and you spot an issue with the product, but can't isolate the cause. To solve this mystery using the conventional method, you'd have to come up with a hypothesis, hand-pick the factors you wanted to analyze, and spend months examining them.
That's where machine learning comes in.
With machine learning software, you don't have to choose what data you want to analyze. In a matter of hours, the software can examine all 50 parameters affecting your chemical product, diagnose the root cause of the issue, and tell you what to change in order to fix it.
Its role isn't limited to problem-solving. When engineers at Volvo wanted to investigate paint application quality at one Sweden plant, they turned to machine learning software. Using this solution, they were able to identify dozens of complex parameters that affected corrosion—some of which they suspected, others that were totally new to them.
From insights to results
Machine learning isn't just for analyzing data. Its true value lies in using data analytics to guide decision-making, delivering tangible results.
After the Volvo engineers identified the complex causes of corrosion, for instance, they could take action to prevent it. By linking the same machine learning software to their paint-spraying robots, they learned how much paint the robots actually needed to protect each truck and could minimize wasted paint, reducing costs and emissions.
In a recent PwC survey of manufacturers, virtually all respondents named efficiency as their top reason for investing in digital factories. Machine learning can boost production throughput by 20% and deliver quality increases as high as 35%, according to Deloitte. After moving to a smart factory, Harley-Davidson reduced one plant's operating costs by $200 million and sliced a fixed 21-day production schedule for new orders down to six hours.
In the manufacturing world, there are always trade-offs. Operators are constantly seeking to balance cost and quality, volume and emissions. Machine learning software can provide a concrete answer to these strategic dilemmas. And as factories move more into automation, the software can even link directly to production robots, freeing up engineers for other pressing tasks.
In the factory of the future, smart connectivity will be crucial. Even today, the COVID-19 pandemic highlights the increased need for digitalization. Manufacturers investing in Industry 4.0 tactics today will set themselves apart from the pack.
Berk Birand is the founder and CEO of Fero Labs, the only industrial process optimization software that uses Explainable Machine Learning to help factories reduce emissions, optimize quality and increase profits. He holds a Ph.D. in electrical engineering and computer science from Columbia University. His academic research focused on optimizing wireless and optical networks with efficient cross-layer algorithms. He holds a patent in IoT systems for resilient fiber-optic networks.
Comments (0)
This post does not have any comments. Be the first to leave a comment below.
Featured Product
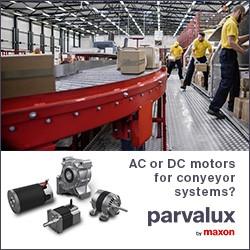