How ML helps manufacturers maintain safer workplaces
Ensuring safe working environments remains a critical challenge for manufacturers across the globe. In the UK manufacturing sector alone, work-related illnesses and injuries cause at least £1 billion of annual financial loss, according to the 2023 HSE Report.
Adopting the right digital tech enables manufacturers to improve workplace safety and health – 100% of respondents to Sphera’s Process Safety Report 2023 state that technology helps improve process safety or has the potential to do so in the future. Machine learning, with its automation and self-learning capabilities, can be the most useful technology in this regard.
This article covers the concept of machine-learning in manufacturing and highlights four use cases of ML improving workplace safety.
Automating unsafe tasks
Companies can enhance workplace safety by automating dangerous manufacturing tasks with the help of ML solutions. In particular, manufacturers can implement ML-powered collaborative robots (cobots), which can assist human workers and learn throughout the joint work process, perfecting their skills.
For example, such cobots can help companies improve arc welding, a process that typically involves heat stress, electric shock, and other safety risks. Such a robot can perform this task based on a predefined arc welding pattern with little or no human intervention, reducing the risks for human operators.
Equipped with ML algorithms, a cobot can also analyze data about its welding environment and quickly adapt to any changes in working conditions. It can automatically switch between different welding patterns (such as butt joint or lap joint welding), which improves the quality of welds and enhances overall production efficiency.
Another task that can be assigned to an ML-powered cobot is packaging and palletizing. A cobot can prepare hazardous materials and goods (even in a noisy or gas-filled work area) for transportation, reducing risks for human workers. An ML-powered cobot can also learn on the fly to perform packaging and palletizing faster and more accurately.
Streamlining equipment and facility maintenance
Many types of equipment, such as generators, electrical installations, or boilers, have an increased risk of breakdown. At best, such a failure can shorten the overall equipment life cycle or increase production downtime. In worst-case scenarios, a breakdown can harm the health and life of manufacturing workers.
Manufacturers can reduce the number of breakdowns and, consequently, reduce safety risks by timely running preventative maintenance. Predictive maintenance solutions equipped with machine learning capabilities can considerably streamline this time-consuming process.
This tool can quickly analyze the wealth of information about equipment downtime events and other equipment-related data (such as average equipment utilization rate, operating conditions, or mechanical characteristics) to generate accurate maintenance plans. By following these plans, a manufacturer can minimize the risk of equipment breakdowns and failures.
Improving the retention of experienced employees
52% of respondents of previously mentioned Sphera’s report consider losing experienced personnel a critical factor increasing workplace safety risks. Thus, manufacturers need to reduce experienced employee churn, and machine learning technology can come in handy in this regard.
As an option, manufacturers can equip their human resource management (HRM) system with ML algorithms to analyze employee turnover in-depth based on employee surveys, performance reviews, and information about their age, salary, and length of service.
Dedicated machine learning algorithms can identify far more correlations and patterns in this data than HR specialists, identifying various hidden factors influencing staff turnover like job dissatisfaction and professional burnout. Based on the results of the analysis, a company’s HR team can define measures that would help reduce employee churn.
Improving manufacturing cyber security
In 2017, a group of hackers used malware called Triton to seize control of the security systems of a petrochemical plant in Saudi Arabia. The attackers were able to take over the plant’s physical controllers and related software remotely, which almost led to a disaster and many casualties.
Hopefully, the hack was identified due to a bug in the malicious code. This allowed employees to timely stop the plant’s work and thereby avoid an accident.
Companies can mitigate such risks by implementing ML-powered cybersecurity systems to process large amounts of disparate data (including web traffic or cybersecurity logs) for automated real-time cybersecurity monitoring.
Such security systems can detect anomalies in data and quickly identify cyber-attacks. Depending on the type and intensity of the attack, such an ML system can notify the IT security team, initiate pressure relief mechanisms, or completely shut down the plant and thereby prevent a disaster.
Final thoughts
Ensuring employee safety has always been one of the most critical tasks for manufacturers. Nowadays, more and more manufacturers are implementing machine learning, a technology providing advanced automation and self-learning capabilities, to handle various aspects of plant safety.
With ML solutions in place, companies can automate unsafe and risky tasks, such as arc welding and pelletizing of hazardous materials and goods. In addition, ML can help a manufacturer streamline equipment maintenance, improve cybersecurity, and decrease the churn of its most experienced employees, which can also result in enhanced workplace safety.
However, implementing the technology can be too complex for manufacturers without solid ML expertise in-house. Fortunately, companies can bridge this gap by turning to third-party ML consultants who can help a manufacturer develop the required ML-powered tools and train ML models.
Comments (0)
This post does not have any comments. Be the first to leave a comment below.
Featured Product
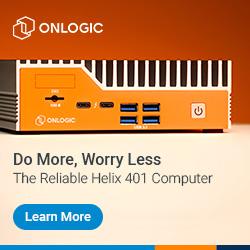